Generative Artificial Intelligence (Generative AI) represents a cutting-edge field within the broader spectrum of artificial intelligence. Unlike traditional AI models that focus on classification or prediction tasks, generative models are designed to create new, original content. This transformative technology has rapidly evolved in recent years, demonstrating its potential across various domains such as image generation, text synthesis, and even music composition. Generative AI, a subfield of artificial intelligence, has emerged as a transformative force, blurring the lines between human and machine creativity. Unlike traditional AI models that focus on analyzing and classifying data, generative AI takes a leap forward, venturing into the realm of content creation. In this article, we will delve into the intricacies of Generative AI, exploring its underlying principles, applications, challenges, and the impact it has on our technological landscape.
What is Generative AI?
Generative AI refers to a category of artificial intelligence that focuses on creating or generating new content, data, or information rather than just analyzing or processing existing data. Unlike traditional AI systems that operate based on predefined rules or explicit instructions, generative AI employs advanced algorithms, often based on neural networks, to learn patterns from large datasets and generate novel outputs.
One key aspect of generative AI is its ability to produce content that was not explicitly present in the training data. This includes generating realistic images, text, music, or other forms of creative output. Notable examples of generative AI include language models like GPT-3 (Generative Pre-trained Transformer 3) and image generation models like DALL-E, Stable Diffusion.
Imagine a world where you can conjure up new ideas, not just consume existing ones. Generative AI empowers you to do just that. It’s a type of AI that can generate entirely new content, from text and images to music and code. Think of it as a digital artist, a tireless composer, or an inventive writer, fueled by data and algorithms.
Generative AI can be used in various applications, such as content creation, art generation, language translation, and even in simulating realistic environments for virtual reality. However, ethical considerations, such as the potential for misuse, bias in generated content, and the need for responsible deployment, are crucial aspects that researchers and developers must address as generative AI continues to advance.
How does Generative AI work?
Generative AI operates on the principles of machine learning, particularly using neural networks, to generate new and often realistic content. The underlying mechanism can vary based on the specific architecture or model being employed, but here’s a general overview of how generative AI typically works:
- Data Collection and Preprocessing:
- Generative AI models require large datasets to learn patterns and features. This data could be anything from images and text to audio or other forms of information.
- The data is preprocessed to ensure that it is in a suitable format for training. This may involve tasks like normalization, cleaning, and encoding.
- Architecture Choice:
- Generative AI models often use neural networks, with specific architectures designed for different types of data and tasks. Common architectures include Variational Autoencoders (VAEs), Generative Adversarial Networks (GANs), and Transformer-based models like GPT (Generative Pre-trained Transformer).
- Training:
- During the training phase, the model is exposed to the prepared dataset. The neural network learns to identify patterns, relationships, and features within the data.
- For GANs, there are two main components: a generator and a discriminator. The generator creates new content, and the discriminator evaluates how realistic that content is. The two components are in a continual feedback loop, with the generator improving its output to fool the discriminator.
- Loss Function:
- A loss function is used to quantify the difference between the generated output and the real data. The model adjusts its parameters to minimize this loss, gradually improving its ability to generate realistic content.
- Fine-Tuning:
- Depending on the architecture, there may be additional fine-tuning steps to enhance the model’s performance on specific tasks. This can involve adjusting hyperparameters, modifying the architecture, or employing transfer learning from pre-trained models.
- Generation:
- Once trained, the generative AI model can produce new content by taking random inputs or following specific instructions. For example, in language models like GPT, providing a prompt results in the model generating coherent and contextually relevant text.
- Ethical Considerations:
- Developers need to be mindful of potential biases in the training data and the generated content. Ethical considerations, responsible deployment, and addressing issues like content manipulation are crucial aspects of generative AI development.
Generative AI has found applications in various fields, including art, content creation, language translation, and more. However, continuous research is needed to refine models and address ethical concerns associated with their use.
What is a modality in Generative AI?
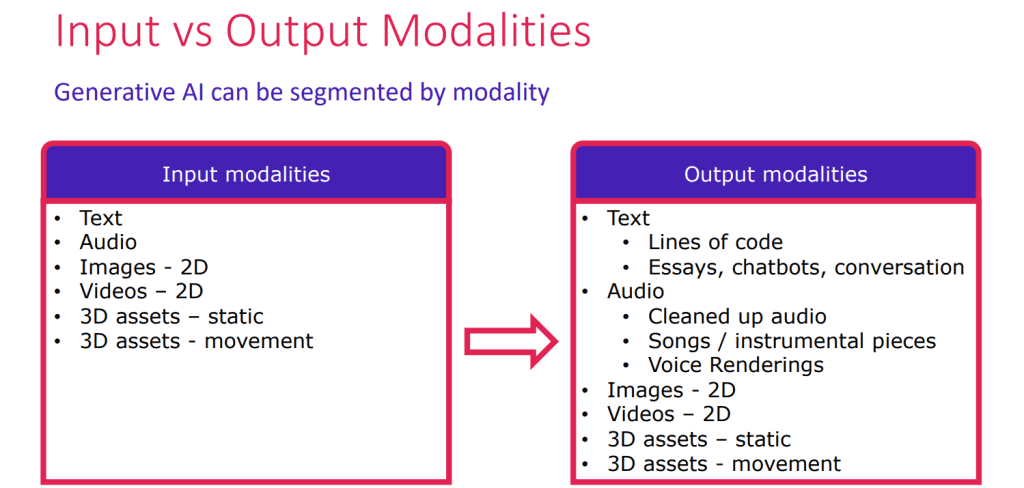
In the context of Generative Artificial Intelligence (Generative AI) and machine learning, the term “modality” refers to a particular mode or type of data or information. It is essentially a way in which information is presented or represented. Different modalities represent distinct types of data, and they can include various forms such as:
- Text Modality:
- Involves textual data, such as written language or documents.
- Image Modality:
- Involves visual data, such as pictures, photographs, or other graphical representations.
- Audio Modality:
- Involves sound data, including speech, music, or other auditory information.
- Video Modality:
- Involves sequences of images and audio, creating a moving visual representation.
- Sensor Modality:
- Involves data from sensors, such as those measuring temperature, pressure, or other physical quantities.
- Modalities in Multimodal Systems:
- When different types of data are combined, it is referred to as a multimodal system. For example, a system that processes both text and images is dealing with multiple modalities.
In the context of Generative AI models, the term “multimodal” is often used when models are designed to handle and integrate information from multiple modalities. For instance, a multimodal model might be capable of understanding both text and images, allowing it to perform tasks that involve a combination of textual and visual information.
Understanding and processing information from different modalities are crucial in various Generative AI applications, such as natural language processing, computer vision, and audio analysis. Developing models that can effectively handle multiple modalities is an active area of research in the field of artificial intelligence.
Notable Players: Innovators in Generative AI
While cutting-edge algorithms and code underpin the remarkable advances in generative AI, creativity comes in many forms. Let’s explore two inspiring examples pushing the boundaries beyond lines of code and into the realms of art and data expression.
DALL-E 2 & Stable Diffusion: Titans of Text-to-Image using Generative AI
These two models have sparked a creative revolution, transforming mere words into vivid, photorealistic images. DALL-E 2’s uncanny ability to translate complex concepts into visual masterpieces, from surreal landscapes to hyperrealistic portraits, has garnered widespread acclaim. Meanwhile, Stable Diffusion democratizes the process, offering an open-source alternative that empowers countless artists and enthusiasts to explore the endless possibilities of text-to-image generation.
Refik Anadol Studios: Painting with Data using Generative AI
Refik Anadol Studios stands out as a pioneer in utilizing generative AI to create a new artform. By harnessing data as pigments, the studio explores the intersection of data and aesthetics, giving rise to mesmerizing visual experiences. Their work exemplifies the transformative potential of generative AI in shaping entirely novel and immersive forms of artistic expression.
Redefining the meaning of “pixel art,” Refik Anadol Studios weaves magic with data, breathing life into numbers and statistics. Their immersive installations transform massive datasets like weather patterns or brain activity into mesmerizing symphonies of light and movement. Each project feels like a portal into the invisible, prompting viewers to contemplate the hidden beauty and poetry within the raw data that surrounds us.
Generative AI Case Study: Video Synopsis Generator
In the age of information overload, where video content bombards us like an endless scroll, finding time to sift through hours of footage can feel like an Olympic feat. Enter the Video Synopsis Generator – a technological knight in shining armor poised to rescue us from the clutches of indecision and time scarcity.
A Video Synopsis Generator is an innovative technology that condenses and summarizes lengthy video footage into a concise and comprehensive visual summary. This tool is designed to efficiently process and distill the essential content from extended video sequences, providing a quick overview of the key events, objects, and activities captured in the footage.
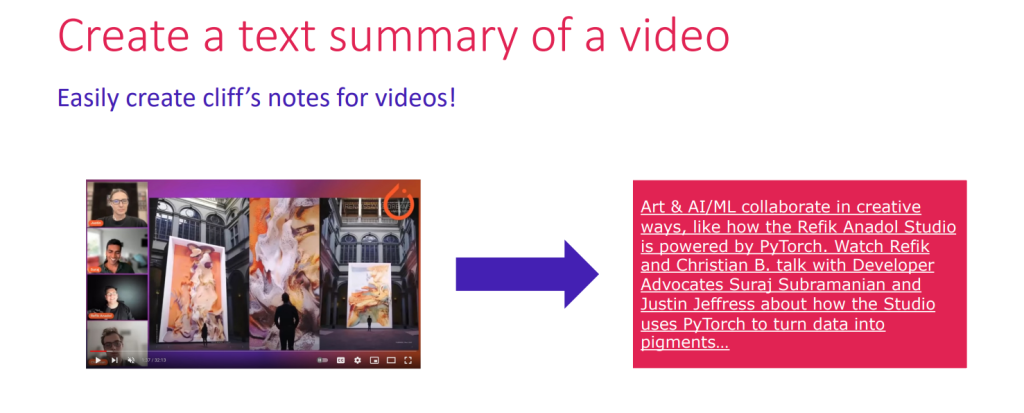
The primary goal of a Video Synopsis Generator is to save time and enhance efficiency in video analysis. By automatically extracting salient information from hours of video content, it allows users to rapidly grasp the core elements without the need to watch the entire footage. This is particularly valuable in surveillance, forensic investigations, and content review scenarios where large volumes of video data need to be analyzed promptly.
The process involves the use of advanced computer vision and machine learning algorithms. These algorithms identify important scenes, objects, and actions within the video, creating a condensed visual representation often in the form of a timeline or a series of keyframes. The resulting video synopsis provides a snapshot of the entire video, highlighting critical moments and aiding in the identification of relevant information.
Applications of Video Synopsis Generators extend beyond security and law enforcement. They can be beneficial in media and entertainment for quick content review, in research for analyzing experiments or observations, and in various industries for monitoring processes and activities.
The efficiency and accuracy of Video Synopsis Generators contribute to improved decision-making by enabling users to quickly assess the content of extensive video archives. As technology continues to advance, these generators are likely to play a crucial role in streamlining video analysis workflows and making video content more accessible and manageable across different domains.
Anatomy of the video summarizer
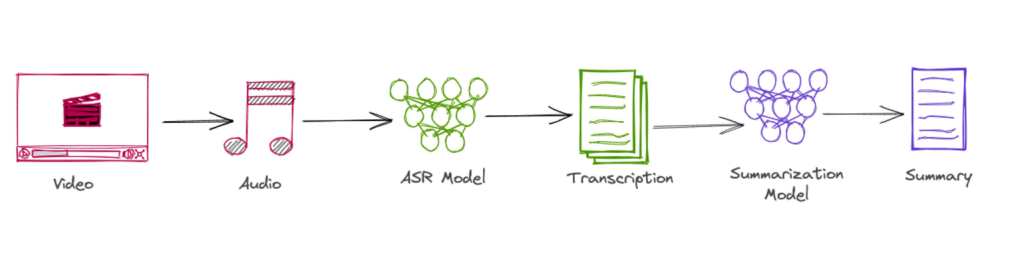
The Anatomy of the Video Summarizer delineates the intricate process through which raw video content transforms into a concise and informative text summary. This multi-step procedure involves the conversion of visual and auditory elements into a textual representation that captures the essence of the video’s content.
- Video Input:
- The process begins with the input of a video, which may contain a diverse array of scenes, objects, and actions. This raw visual data serves as the foundation for the subsequent steps in the summarization pipeline.
- Audio Extraction:
- The video’s audio component is extracted to preserve and utilize auditory cues present in the footage. This step is crucial for a comprehensive understanding of the content, as it enables the system to capture spoken words, ambient sounds, and other audio elements.
- Automatic Speech Recognition (ASR) Model:
- The extracted audio undergoes analysis by an Automatic Speech Recognition (ASR) model. This sophisticated technology translates spoken language into text, converting the auditory information within the video into a textual format that can be further processed.
- Transcription:
- The output of the ASR model results in a transcription—a textual representation of the spoken words and other audio elements present in the video. This transcription acts as a bridge between the audio and summarization phases, providing a structured format for subsequent analysis.
- Summarization Algorithm:
- The transcription text is then fed into a summarization algorithm designed to distill the most pertinent information from the entire video content. This algorithm assesses the importance of various segments, considering factors such as keywords, sentiments, and contextual relevance.
- Text Summary Output:
- The final output of the video summarizer is a concise text summary that encapsulates the key elements of the video. This summary serves as a condensed representation of the original content, providing users with an efficient and informative overview without the need to watch the entire video.
This comprehensive process, from video to text summary, showcases the synergy of advanced technologies such as ASR and summarization algorithms. The Video Summarizer not only accelerates content review but also makes vast amounts of video data more accessible and manageable, finding applications in diverse fields such as research, media, and surveillance.
Extract audio from video
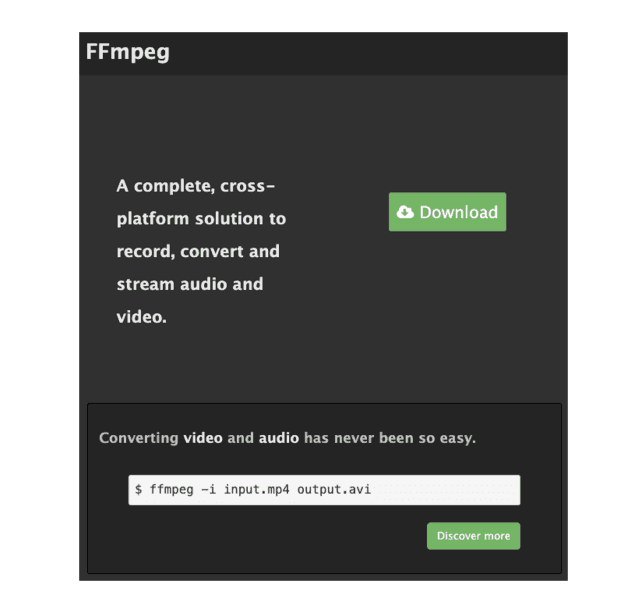
The process of extracting audio from a video involves utilizing specialized tools, such as FFMPEG, to separate the audio component from the visual content. This extraction facilitates the independent use of audio data or further analysis. Here’s an overview of the steps involved:
FFMPEG – Multimedia Handling Suite
- FFMPEG stands out as a comprehensive suite of libraries and programs designed for handling a variety of multimedia files, including video and audio. It provides a versatile set of tools for manipulating, converting, and processing multimedia content.
Command-Line Tool
- FFMPEG is primarily a command-line tool, requiring users to input specific commands for desired operations. This command-line interface allows for flexibility and customization in handling multimedia files.
Python Integration
- While FFMPEG is a command-line tool, it can seamlessly integrate with Python environments such as Jupyter notebooks. Using the exclamation mark (!) as a prefix in a Python cell allows for the execution of command-line instructions, making FFMPEG accessible and executable directly from Python notebooks.
Extraction Command
- To extract audio from a video using FFMPEG, a command similar to the following can be employed in a Python notebook:
!ffmpeg -i input.mp4 output.avi
This command specifies the input video file (input.mp4) and the desired output file format (output.avi).
Conversion Process
- The
-i
flag in the command denotes the input file, and FFMPEG automatically recognizes the format based on the file extension. The extraction process separates the audio content from the video, producing a file in the specified output format.
Output
- The result of the extraction process is a standalone audio file (output.avi in the given example), which can be further analyzed, processed, or used independently of the original video.
The ability to extract audio from a video using FFMPEG provides users with flexibility in working with multimedia content. Whether for audio analysis, editing, or other applications, this process enhances the versatility of multimedia data in various contexts, including programming environments like Python notebooks.
Automatic Speech Recognition (ASR)
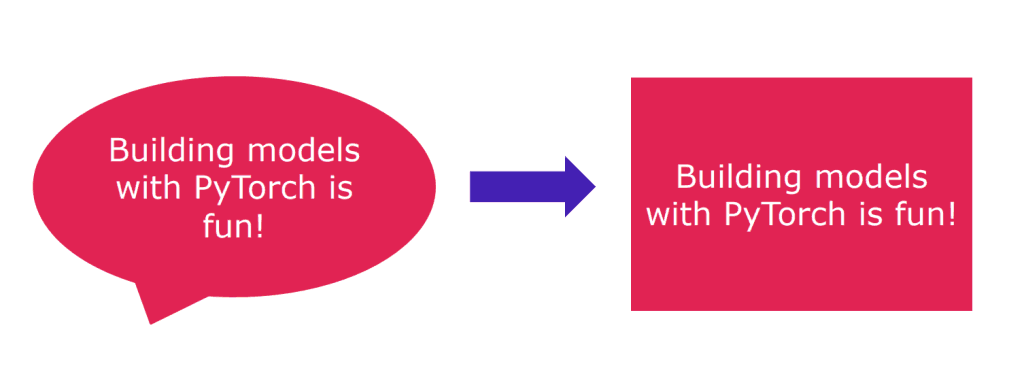
Automatic Speech Recognition (ASR) is a technology that converts spoken language into written text. This process involves intricate algorithms and models designed to analyze audio signals and transcribe them into textual representations. Here’s an overview of the key components and steps involved in Automatic Speech Recognition:
- Audio Input:
- ASR begins with an audio input, typically in the form of spoken words or phrases. This audio can be sourced from various mediums, including recorded speech, live conversations, or any form of spoken communication.
- Feature Extraction:
- The audio signal undergoes feature extraction, a process where relevant characteristics, such as frequency components, are identified. Mel-frequency cepstral coefficients (MFCCs) are commonly used features in ASR systems.
- Acoustic Modeling:
- Acoustic models form a crucial part of ASR systems. These models are trained to associate acoustic features extracted from the audio signal with phonemes or sub-word units. Deep neural networks are often employed for this task, capturing complex patterns in the audio data.
- Language Modeling:
- Language models complement acoustic models by incorporating linguistic context. They help the system predict the most likely word sequences based on the audio input. N-gram models and neural language models contribute to this linguistic aspect.
- Decoding:
- During decoding, the ASR system aligns the acoustic and language models to find the most probable word sequence that corresponds to the input audio. Various algorithms, such as Viterbi decoding, are applied to determine the optimal transcription.
- Transcription Output:
- The final output of the ASR process is a textual transcription of the spoken words in the input audio. This transcription can be in the form of raw text or a sequence of words, depending on the design of the ASR system.
- Post-Processing (Optional):
- In some cases, post-processing steps may be applied to refine the transcription. This could include language model-based corrections, context-aware adjustments, or other techniques to enhance the accuracy of the output.
ASR finds applications in various domains, including voice assistants, transcription services, voice-controlled systems, and accessibility tools. Its development has been greatly influenced by advancements in deep learning, leading to more robust and accurate speech recognition systems. The continuous improvement of ASR technology contributes to its widespread use in making spoken language accessible and actionable in diverse contexts.
Text summarization
Text summarization is a computational process that involves generating a concise and accurate summary of a given input text. Over time, the evolution of Natural Language Processing (NLP) architectures has played a significant role in enhancing the effectiveness of text summarization. Here’s an overview of the key aspects involved in text summarization:
- Objective:
- The primary objective of text summarization is to distill the essential information from a longer piece of text while preserving its core meaning. This is crucial for quickly conveying the key points without the need to read the entire document.
- Historical Context – Recurrent Neural Networks (RNNs):
- In the earlier stages of NLP, recurrent neural networks (RNNs) were commonly used for text summarization. However, RNNs had limitations in capturing long-range dependencies, affecting their ability to generate coherent and contextually rich summaries.
- Modern Approach – Transformer-Based Models:
- Modern NLP models, particularly transformer-based architectures like BERT (Bidirectional Encoder Representations from Transformers) and GPT (Generative Pre-trained Transformer), have demonstrated superior performance in text summarization. Transformers excel in capturing contextual relationships across words and have become the backbone of state-of-the-art NLP applications.
- Specialized Summarization Models:
- Summarization models are specialized language models that have been fine-tuned specifically for the task of summary generation. They leverage large datasets, such as CNN Dailymail and Amazon reviews, to learn the nuances of summarizing diverse content.
- Training on Summarization Datasets:
- To enhance their summarization capabilities, models undergo training on datasets containing pairs of original text and corresponding summaries. This process allows the model to learn how to distill crucial information and produce coherent and concise summaries.
- Input Length Constraints:
- Summarization models often have limitations on the length of the input they can effectively process. This constraint is typically expressed in terms of the number of tokens constituting the input. Managing input length is crucial for maintaining computational efficiency and model performance.
In short, text summarization has evolved from relying on RNNs to leveraging transformer-based models, leading to substantial improvements in the quality of generated summaries. These modern architectures, fine-tuned for summarization tasks, play a pivotal role in various applications, including content summarization, news aggregation, and information retrieval.
Tokenization

Tokenization is a fundamental process in Natural Language Processing (NLP) that involves breaking down a large body of text into smaller, more manageable units known as tokens. Tokens can represent individual words, phrases, or even entire sentences, depending on the level of granularity required for a particular NLP task. Here’s an overview of key aspects related to tokenization:
- Definition:
- Tokenization is the process of segmenting a continuous text into discrete units, or tokens. These tokens serve as the building blocks for subsequent analysis in NLP tasks.
- Types of Tokens:
- Tokens can take various forms, including individual words, phrases, or complete sentences. The choice of tokenization granularity depends on the specific requirements of the NLP application.
- Word-Level Tokenization:
- In word-level tokenization, the text is divided into individual words. Each word becomes a separate token, enabling the analysis of the text at the finest level of detail.
- Phrase-Level Tokenization:
- For certain tasks, tokenization may occur at the phrase level, where groups of words are treated as a single unit. This approach allows for the extraction of meaningful multi-word expressions.
- Sentence-Level Tokenization:
- In sentence-level tokenization, the text is segmented into complete sentences. Each sentence then becomes a distinct token, facilitating tasks that require understanding at the sentence level.
- Purpose of Tokenization:
- The primary purpose of tokenization is to make the text more manageable and easier to process for subsequent NLP tasks. Breaking down the text into smaller units simplifies the analysis and allows for a more granular understanding of the content.
- Preprocessing Step:
- Tokenization is often a crucial preprocessing step in NLP pipelines. It sets the foundation for tasks such as sentiment analysis, machine translation, and named entity recognition by organizing the input text into meaningful units.
- Challenges in Tokenization:
- Despite its importance, tokenization can pose challenges, especially in languages with complex word structures or in tasks requiring specialized tokenization rules. Techniques like subword tokenization and byte pair encoding (BPE) are employed to address these challenges.
Finally, tokenization is a pivotal process in NLP that transforms raw text into structured units, facilitating effective language analysis. Its versatility allows for adaptation to various levels of linguistic granularity, making it a fundamental step in the preprocessing of textual data for a wide range of NLP applications.
Conclusion
Generative AI represents a paradigm shift in artificial intelligence, empowering machines to create original content across various domains. As technology advances, it is crucial to address ethical concerns, biases, and challenges associated with this transformative field. The ongoing evolution of generative AI promises to reshape industries, foster innovation, and raise new questions about the intersection of technology and humanity. As we navigate this frontier of innovation, a thoughtful and ethical approach will be key to harnessing the full potential of generative AI for the benefit of society.
As generative AI technology continues to evolve, we can expect even more mind-blowing applications to emerge. Imagine a world where we can collaborate with AI to create art, design cities, and compose symphonies. The possibilities are truly endless.
Generative AI is not just a technological marvel; it’s a paradigm shift in how we think about creativity. It challenges us to redefine the boundaries between human and machine, and to embrace the possibilities of a future where imagination knows no bounds.